The course aims to bring the students knowledge about key concepts that form artificial intelligence (AI), and their applications within the field of sustainable energy engineering. With focus on machine learning (ML), the students will be given insight in the fundamental theory and algorithms that shape ML models, as well as how to select method and data based on various applications. The course also introduces potential implications for energy businesses, as well as ethical aspects of using artificial intelligence. The students will learn to manage data for the purpose of ML applications as well as to create, integrate and use ML for analysis and design in an energy context. Based on the course content, students are expected to describe advantages and limitations for AI applications in the energy sector, as well as discuss trends and potential risks connected to the subject.
MJ2507 AI applications in Sustainable Energy Engineering 6.0 credits
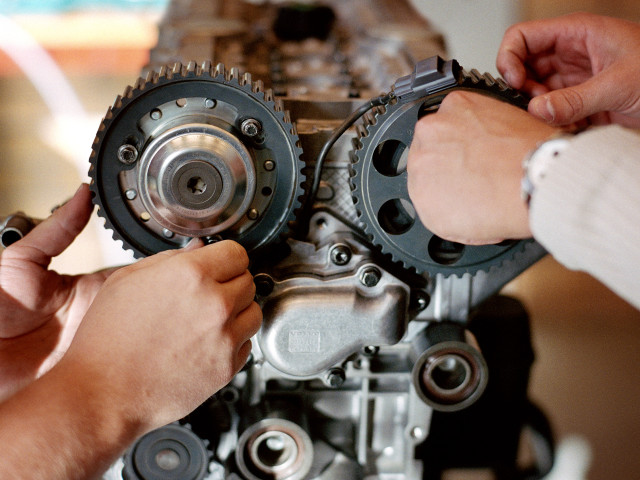
Choose semester and course offering
Choose semester and course offering to see current information and more about the course, such as course syllabus, study period, and application information.
Application
For course offering
Autumn 2024 Start 26 Aug 2024 programme students
Application code
50380
Content and learning outcomes
Course contents
Intended learning outcomes
The course aims to providing the students with fundamental background and tools for understanding the fundamentals in artificial intelligence and using machine learning in applications within sustainable energy engineering.
After completing the course, the student will be able to
- Explain and describe the background and areas of use for artificial intelligence with focus on machine learning, including the main characteristics for common techniques.
- Explain and describe the method for developing a ML model, including selection of technique, treatment of data, design, model evaluation and model improvement.
- Design a working machine learning model aimed to optimize design or operation in a case related to energy, including assessment of model’s performance according to common evaluation steps and critical comparison to a non-AI approach.
- Identify existing AI trends in the energy sector including companies and implications in their business
- Demonstrate an insight to advantages, limitations, and risks connected to AI’s role in society, and specifically from the energy sector perspective.
Literature and preparations
Specific prerequisites
Knowledge in renewable energy corresponding to course MJ2411 "Renewable energy" 6 credits
Recommended prerequisites
Knowledge in Matlab or Pyhton.
Equipment
Literature
Examination and completion
If the course is discontinued, students may request to be examined during the following two academic years.
Grading scale
Examination
- INL1 - Home Ass', 1.5 credits, grading scale: P, F
- KON1 - Quiz, 1.5 credits, grading scale: A, B, C, D, E, FX, F
- PRO1 - Project, 3.0 credits, grading scale: A, B, C, D, E, FX, F
Based on recommendation from KTH’s coordinator for disabilities, the examiner will decide how to adapt an examination for students with documented disability.
The examiner may apply another examination format when re-examining individual students.
Opportunity to complete the requirements via supplementary examination
Opportunity to raise an approved grade via renewed examination
Examiner
Ethical approach
- All members of a group are responsible for the group's work.
- In any assessment, every student shall honestly disclose any help received and sources used.
- In an oral assessment, every student shall be able to present and answer questions about the entire assignment and solution.