- Computational foundations
- Biological foundations
- Linear models
- Deep neural networks
- Kernel methods, trees, & forests
- Unsupervised learning
- Society, ethics, and broader impacts
CB206V Machine Learning in Biotechnology 7.5 credits
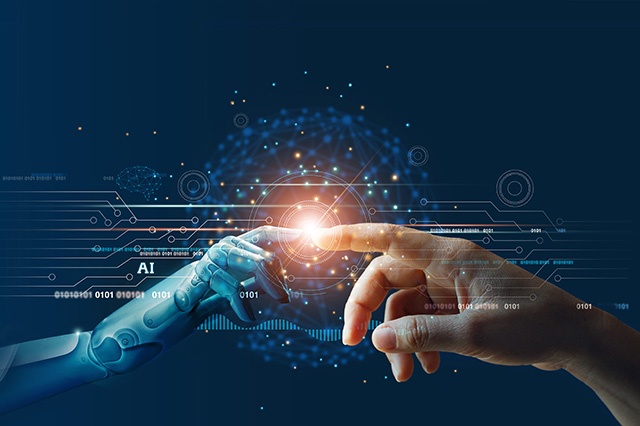
Welcome to a course that provides an introduction to machine learning and how deep learning strategies are used and developed in biotechnological applications. The course covers the foundations of data-based modeling and builds on this topic with machine learning modules relevant to genomics, protein sciences, and imaging. The course contains lectures and hands-on programming exercises, introducing distinct machine learning modalities. We cover supervised learning and unsupervised learning, and consider challenges and possibilities in applying these methods to biological data. At the end of the course, the participants are expected to have a broad knowledge of different machine learning techniques, concrete understanding of how to implement data based models, and how these tools can be used in the context of biotechnology. After the course, the participants are expected to be well-positioned to integrate machine learning techniques into their own research.
Choose semester and course offering to see current information and more about the course, such as course syllabus, study period, and application information.
Content and learning outcomes
Course contents
Intended learning outcomes
After completion of the course, the students shall have knowledge to:
- Explain the concepts of artificial intelligence/machine learning, including supervised and unsupervised learning, deep neural networks, and optimization.
- Apply machine learning algorithms to a range of data types and domains, including images and genomic data, to solve real-world problems related to biotechnology.
- Evaluate the performance of machine learning models using appropriate metrics and techniques, and interpret the results to draw meaningful conclusions.
- Identify ethical and societal implications of machine learning, including issues related to fairness, privacy, and accountability.
- Communicate machine learning concepts and results effectively to both technical and non-technical audiences, using appropriate visualizations and language.
- Explain how machine learning could begin to be integrated into their own research.
Literature and preparations
Specific prerequisites
Completed degree project 15 credits, 20 credits in biotechnology, genomics, bio(medical) sciences, data sciences, or biostatistics, 6 credits in mathematics, and English B/6.
Recommended prerequisites
Equipment
Literature
Probabilistic Machine Learning: an Introduction (Course Book)
Selected articles and on-line material.
Examination and completion
If the course is discontinued, students may request to be examined during the following two academic years.
Grading scale
Examination
- PRO1 - Group project, 2.5 credits, grading scale: P, F
- SEM1 - Group work and seminar presentation, 1.0 credits, grading scale: P, F
- TEN1 - Oral exam, 4.0 credits, grading scale: P, F
Based on recommendation from KTH’s coordinator for disabilities, the examiner will decide how to adapt an examination for students with documented disability.
The examiner may apply another examination format when re-examining individual students.
Opportunity to complete the requirements via supplementary examination
Opportunity to raise an approved grade via renewed examination
Examiner
Ethical approach
- All members of a group are responsible for the group's work.
- In any assessment, every student shall honestly disclose any help received and sources used.
- In an oral assessment, every student shall be able to present and answer questions about the entire assignment and solution.