- Basic definitions in graph theory, strong and weak bands, grade distribution and clustering measurements.
- Erdos-Renyi, Wats-Strogatz, ccnfiguration models, the effect of a "small world".
- Random walks in graphs, Page Rank.
- Graph clustering, identification of "communities".
- The algorithm "Label Propagation", link prediction.
- Basics of machine learning of graph representations.
ID2211 Data Mining, Basic Course 7.5 credits
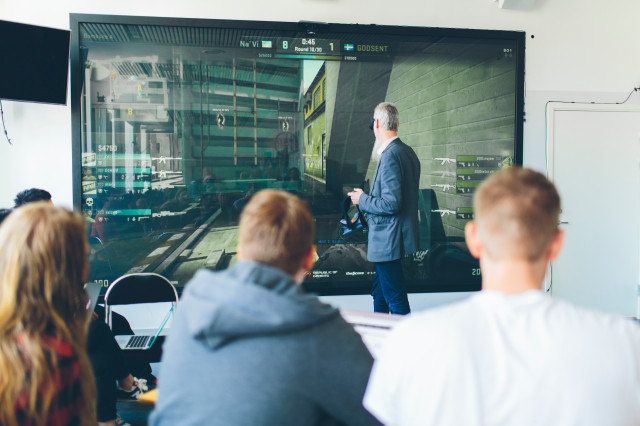
In the course, the foundations of data mining is studied, with a special focus on information network analysis and mining.
About course offering
For course offering
Spring 2025 Start 17 Mar 2025 programme students
Target group
Open to all master's programmes as long as it can be included in your programme.
Part of programme
Master's Programme, ICT Innovation, åk 1, CLNI, Conditionally Elective
Master's Programme, ICT Innovation, åk 1, CLNS, Conditionally Elective
Periods
P4 (7.5 hp)Duration
Pace of study
50%
Form of study
Normal Daytime
Language of instruction
English
Course location
KTH Kista
Number of places
Places are not limited
Planned modular schedule
Course memo
Course memo is not publishedSchedule
Schedule is not publishedApplication
For course offering
Spring 2025 Start 17 Mar 2025 programme students
Application code
60693
Contact
For course offering
Spring 2025 Start 17 Mar 2025 programme students
Examiner
No information insertedCourse coordinator
No information insertedTeachers
No information insertedContent and learning outcomes
Course contents
Intended learning outcomes
After passing the course, the student shall be able to
- explain different fundamental concepts and algorithms in data mining and basic technologies for analysis and extraction in information networks (for example the fundamental concepts in graph theory, network models, algorithms left graph clustering, identification of "communities", "Label Propagation", link prediction, etc)
- analyse, choose, use, and evaluate technologies for data mining that is based on the above concepts and explore and implement the existing data mining algorithms independently
- communicate findings, results and ideas with clear and formal language.
Literature and preparations
Specific prerequisites
Recommended prerequisites
Familiarity with the basic probability theory, linear algebra as well as ability to write a non-trivial computer program.
Equipment
Literature
Examination and completion
If the course is discontinued, students may request to be examined during the following two academic years.
Grading scale
Examination
- PRO1 - Project, 3.0 credits, grading scale: P, F
- TEN1 - Examination, 4.5 credits, grading scale: A, B, C, D, E, FX, F
Based on recommendation from KTH’s coordinator for disabilities, the examiner will decide how to adapt an examination for students with documented disability.
The examiner may apply another examination format when re-examining individual students.
The exam is written.
Opportunity to complete the requirements via supplementary examination
Opportunity to raise an approved grade via renewed examination
Examiner
Ethical approach
- All members of a group are responsible for the group's work.
- In any assessment, every student shall honestly disclose any help received and sources used.
- In an oral assessment, every student shall be able to present and answer questions about the entire assignment and solution.
Further information
Course room in Canvas
Offered by
Main field of study
Education cycle
Add-on studies
Supplementary information
In this course, the EECS code of honor applies, see: http://www.kth.se/en/eecs/utbildning/hederskodex.