- Introduction to Data Mining
- Frequent Itemsets
- Finding Similar Items
- Clustering
- Recommendation Systems
- Mining Data Streams
- Dimensionality Reduction
- Large-Scale Machine Learning
ID2222 Data Mining 7.5 credits
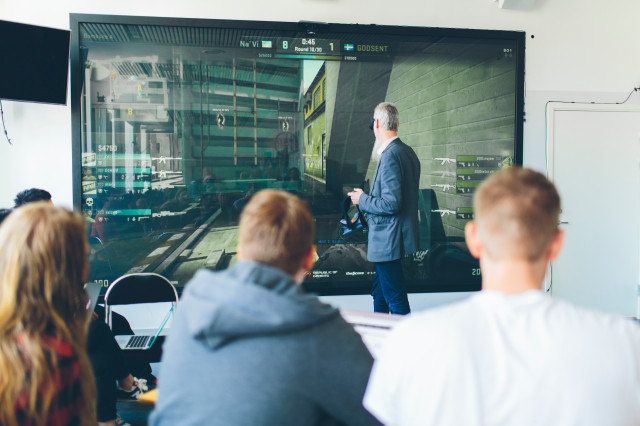
About course offering
For course offering
Autumn 2024 Start 28 Oct 2024 programme students
Target group
Open to all programmes as long as it can be included in your programme.
Part of programme
Master's Programme, ICT Innovation, åk 2, CLNI, Recommended
Master's Programme, ICT Innovation, åk 2, CLNS, Recommended
Master's Programme, ICT Innovation, åk 2, DASC, Mandatory
Master's Programme, ICT Innovation, åk 2, DASE, Mandatory
Master's Programme, Industrial Engineering and Management, åk 1, MAIG, Conditionally Elective
Master's Programme, Machine Learning, åk 1, Conditionally Elective
Master's Programme, Machine Learning, åk 2, Conditionally Elective
Master's Programme, Software Engineering of Distributed Systems, åk 1, DASC, Conditionally Elective
Master's Programme, Software Engineering of Distributed Systems, åk 1, PVT, Conditionally Elective
Periods
P2 (7.5 hp)Duration
Pace of study
50%
Form of study
Normal Daytime
Language of instruction
English
Course location
KTH Kista
Number of places
Min: 25
Planned modular schedule
Course memo
Course memo is not publishedSchedule
Link to scheduleApplication
For course offering
Autumn 2024 Start 28 Oct 2024 programme students
Application code
50247
Contact
For course offering
Autumn 2024 Start 28 Oct 2024 programme students
Contact
Sarunas Girdzijauskas
Examiner
No information insertedCourse coordinator
No information insertedTeachers
No information insertedContent and learning outcomes
Course contents
Intended learning outcomes
The course studies fundamentals of data mining, data stream processing, and machine learning algorithms for analyzing very large amounts of data. We will use big data processing platforms, such as MapReduce, Spark and Apache Flink, for implementing parallel algorithms, as well as computation systems for data stream processing, such as Storm and InfoSphere.
After this course, students will be able to mine different types of data, e.g., high-dimensional data, graph data, and infinite/never-ending data (data streams); as well as to program and build data-mining applications. They are also expected to know how to solve problems in real-world applications, e.g., recommender systems, association rules, link analysis, and duplicate detection. Moreover, they will master various mathematical techniques, e.g., linear algebra, optimization, and dynamic programming.
Literature and preparations
Specific prerequisites
Recommended prerequisites
Equipment
Literature
The contents of the course are derived from the following two textbooks:
A. Rajaraman and J. D. Ullman, Mining of massive datasets. Cambridge University Press, 2012 (alternative: J. Han, M. Kamber, J. Pei, Data Mining: Concepts and Techniques, 3-rd Ed., Morgan Kaufmann, 2012)
Examination and completion
If the course is discontinued, students may request to be examined during the following two academic years.
Grading scale
Examination
- LAB1 - Programming Assignments, 3.0 credits, grading scale: P, F
- TEN1 - Examination, 4.5 credits, grading scale: A, B, C, D, E, FX, F
Based on recommendation from KTH’s coordinator for disabilities, the examiner will decide how to adapt an examination for students with documented disability.
The examiner may apply another examination format when re-examining individual students.
Written examination. Laboratory tasks.
Opportunity to complete the requirements via supplementary examination
Opportunity to raise an approved grade via renewed examination
Examiner
Ethical approach
- All members of a group are responsible for the group's work.
- In any assessment, every student shall honestly disclose any help received and sources used.
- In an oral assessment, every student shall be able to present and answer questions about the entire assignment and solution.
Further information
Course room in Canvas
Offered by
Main field of study
Education cycle
Add-on studies
Contact
Supplementary information
In this course, the EECS code of honor applies, see: http://www.kth.se/en/eecs/utbildning/hederskodex.