- AI basis for learning machines.
- Statistical learning theory I: Perceptrons and neural networks.
- Statistical learning theory II: The learning problem.
- Machine learning methods.
- Internet psychiatry as a typical case for learning machines.
- Critical perspectives on learning machines.
- Systematic properties of learning machines LM2LM communication and learning in multi agent systems.
- Technological change and the future of learning machines.
- Applications of learning machines.
ID2225 Learning Machines 7.5 credits
This course will be discontinued.
Last planned examination: Spring 2024
Decision to discontinue this course:
The course will be discontinued at the end of the spring semester of 2024 according to Head of School decision: J-2022-1530. Decision date: 2022-06-16
The course was given for the last time in the spring semester of 2021. The last opportunity for examination in the course is given in the spring semester of 2024.
RAP1 and SEM1 modules can be examined according to agreement with the examiner up to and including VT24.
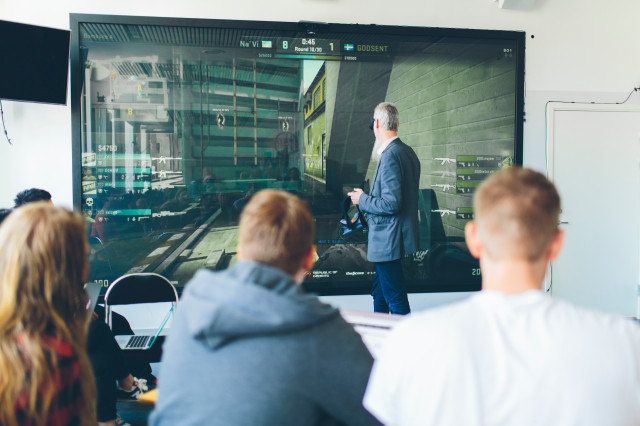
Content and learning outcomes
Course contents
Intended learning outcomes
Having passed the course, the student should be able to:
1. solve problems independently
a. take part in the literature about learning machines and account for their role both historically and today, and in the future, b. relate own models to already existing research and development in the area of learning machines,
2. master abstraction
a. define what a learning machine is and is not,.b. identify relevant concepts and applicable methods and tools,
c. associate critically different relevant concepts and phenomena with learning machines,
d. instrumentalise relevant abstract concepts
3. implement learning machines
a. use tools to build own learning machines, as well as analyse others', b. program, test and critically evaluate own software for learning machines,
c. estimate the correctness and the computational complexity in programs for learning machines.
in order to
- gain knowledge of the different ways in which machine learning methods can be combined to be enclosed in physical or abstract models, so-called learning machines,
- be able to discuss how difficult problems are best solved by means of learning machines,
- be able to evaluate which problems, which overhead costs and which meta learning tools that should be used
- obtain an approach to the subject that admits ethical perspectives in which general artificial intelligence is included.
For higher grades, the student should also be able to
- analyse statistical disturbance factors (confounders), over fitting as well as generalizability in own solutions based on learning machines,
- carry out self critical review of own programming of learning machines including ethical perspectives and sustainability perspectives, as well as to document the same.
- master the meta level through modelling of different solutions based on learning machines, that is to be able to talk about these using adequate terminology.
Literature and preparations
Specific prerequisites
Recommended prerequisites
Equipment
Literature
Examination and completion
If the course is discontinued, students may request to be examined during the following two academic years.
Grading scale
Examination
- RAP1 - Examination report, 4.5 credits, grading scale: A, B, C, D, E, FX, F
- SEM1 - Active participiation in seminars, 3.0 credits, grading scale: P, F
Based on recommendation from KTH’s coordinator for disabilities, the examiner will decide how to adapt an examination for students with documented disability.
The examiner may apply another examination format when re-examining individual students.
Opportunity to complete the requirements via supplementary examination
Opportunity to raise an approved grade via renewed examination
Examiner
Ethical approach
- All members of a group are responsible for the group's work.
- In any assessment, every student shall honestly disclose any help received and sources used.
- In an oral assessment, every student shall be able to present and answer questions about the entire assignment and solution.