This course presents an overview of the most important methods of the modern theory of statistical learning. Topics covered include supervised learning with a focus on classification methods, support vector machines, artificial neural networks, decision trees, boosting, bagging and methods of unsupervised learning with focus on K-means clustering and nearest neighbours. This course focuses primarily on the practical aspects of statistical learning. Computer-aided project work with a variety of datasets forms the essential learning activity.
SF2935 Modern Methods of Statistical Learning 7.5 credits
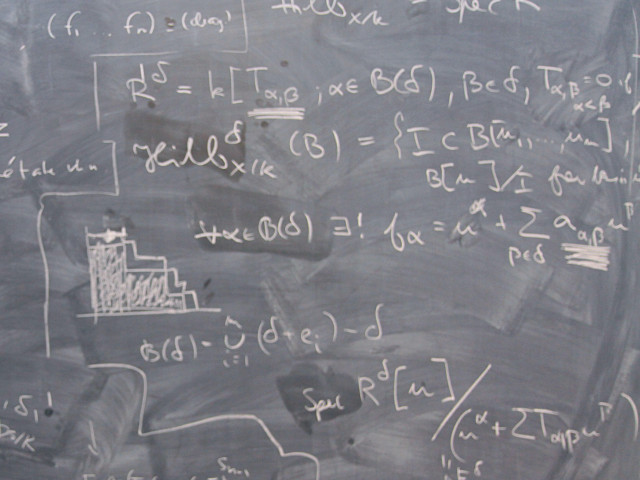
About course offering
For course offering
Autumn 2024 Start 26 Aug 2024 programme students
Target group
Available for all master program students as long as it can be included in your programme.
Part of programme
Master's Programme, Aerospace Engineering, åk 2, Optional
Master's Programme, Aerospace Engineering, åk 2, SYS, Optional
Master's Programme, Applied and Computational Mathematics, åk 1, Optional
Master's Programme, Applied and Computational Mathematics, åk 2, Optional
Master's Programme, Applied and Computational Mathematics, åk 2, DAVE, Mandatory
Master's Programme, Computer Simulations for Science and Engineering, åk 2, Conditionally Elective
Master's Programme, Industrial Engineering and Management, åk 1, FMIB, Conditionally Elective
Master's Programme, Industrial Engineering and Management, åk 1, OSYT, Conditionally Elective
Master's Programme, Information and Network Engineering, åk 2, Recommended
Master's Programme, Information and Network Engineering, åk 2, INF, Recommended
Periods
P1 (7.5 hp)Duration
Pace of study
50%
Form of study
Normal Daytime
Language of instruction
English
Course location
KTH Campus
Number of places
Places are not limited
Planned modular schedule
Course memo
Course memo is not publishedSchedule
Link to scheduleApplication
For course offering
Autumn 2024 Start 26 Aug 2024 programme students
Application code
50503
Contact
For course offering
Autumn 2024 Start 26 Aug 2024 programme students
Contact
Pierre Nyquist (pierren@kth.se)
Examiner
No information insertedCourse coordinator
No information insertedTeachers
No information insertedContent and learning outcomes
Course contents
Intended learning outcomes
For the methods presented in the course, the student shall possess both theoretical and practical understanding of how the methods work, which ones to choose for a given problem and how to implement rudimentary versions of them. Computer-aided projects form an essential learning activity.
To pass the course the student shall be able to
- formulate and apply methods for supervised learning,
- formulate and apply methods for unsupervised learning,
- apply mathematical theory to analysis and explain properties of methods in statistical learning,
- design and implement methods in statistical learning for different tasks.
Literature and preparations
Specific prerequisites
- English B / English 6
- Completed basic course in probability theory and mathematical statistics (SF1918, SF1922 or equivalent).
Recommended prerequisites
Numerical methods (SF1544, SF1545 or similar), differential equations (SF1633, SF1683 or similar), probability and statistics (SF2940 or similar), regression analysis (SF2930 or similar).
Equipment
Literature
Examination and completion
If the course is discontinued, students may request to be examined during the following two academic years.
Grading scale
Examination
- TENA - Examination, 4.5 credits, grading scale: A, B, C, D, E, FX, F
- ÖVN1 - Assignments, 3.0 credits, grading scale: P, F
Based on recommendation from KTH’s coordinator for disabilities, the examiner will decide how to adapt an examination for students with documented disability.
The examiner may apply another examination format when re-examining individual students.
The written exam deals with concepts.
Opportunity to complete the requirements via supplementary examination
Opportunity to raise an approved grade via renewed examination
Examiner
Ethical approach
- All members of a group are responsible for the group's work.
- In any assessment, every student shall honestly disclose any help received and sources used.
- In an oral assessment, every student shall be able to present and answer questions about the entire assignment and solution.