The following fields are treated within the scope of the course: problem-solving with search algorithms, heuristics, knowledge representations (logic), planning, representation of uncertainty and inference (Bayesian networks, HMM), decision theory and utility theory, diction (NLP).
DD2380 Artificial Intelligence 6.0 credits
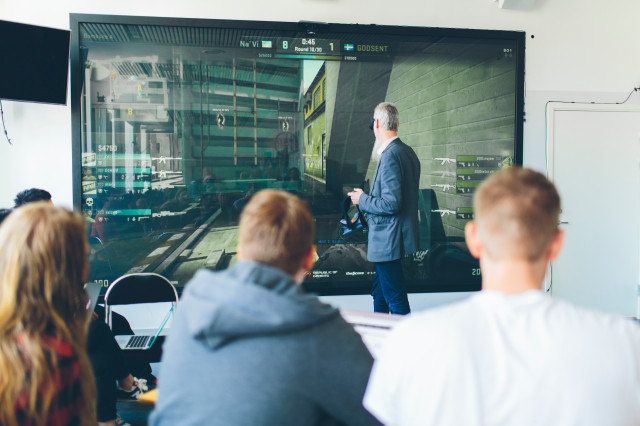
The course gives a broad overview of the problems and methods studied in the field of artificial intelligence.
Choose semester and course offering to see current information and more about the course, such as course syllabus, study period, and application information.
Content and learning outcomes
Course contents
Intended learning outcomes
After passing the course, the student shall be able to
- apply different principles of Artificial Intelligence (AI)
- choose appropriate tools and implement efficient solutions to problems in AI
- integrate tools to design computer programs that show different properties that are expected by an intelligent system
- present, analyse, and entitle an own solution to an AI problem
- reflect on and discuss current social and ethical aspects of AI
in order to be able to
- draw use of methods of artificial intelligence in analysis, design and implementation of computer programs
- contribute to design of an intelligent system in both academic and industrial applications.
Literature and preparations
Specific prerequisites
- Knowledge in algebra and geometry, 7.5 higher education credits, equivalent to completed course SF1624/SF1672.
- Knowledge in one variable calculus, 7.5 higher education credits, equivalent to completed course SF1625/SF1673.
- Knowledge in probability theory and statistics, 6 higher education credits, equivalent to completed course SF1910- SF1924/SF1935.
- Knowledge and skills in programming, 6 higher education credits, equivalent to completed course DD1337/DD1310-DD1319/DD1321/DD1331/DD100N/ID1018.
- Knowledge in algorithms and data structures, at least 6 higher education credits, equivalent to completed course DD1338/DD1320-DD1327/DD2325/ID1020/ID1021.
Active participation in a course offering where the final examination is not yet reported in LADOK is considered equivalent to completion of the course.
Being registered for a course counts as active participation.
The term 'final examination' encompasses both the regular examination and the first re-examination.
Recommended prerequisites
Equipment
Literature
Examination and completion
If the course is discontinued, students may request to be examined during the following two academic years.
Grading scale
Examination
- LAB2 - Laboratory work, 4.0 credits, grading scale: A, B, C, D, E, FX, F
- RAP1 - Report, 0.5 credits, grading scale: P, F
- TENQ - Quiz, 1.5 credits, grading scale: P, F
Based on recommendation from KTH’s coordinator for disabilities, the examiner will decide how to adapt an examination for students with documented disability.
The examiner may apply another examination format when re-examining individual students.
Opportunity to complete the requirements via supplementary examination
Opportunity to raise an approved grade via renewed examination
Examiner
Ethical approach
- All members of a group are responsible for the group's work.
- In any assessment, every student shall honestly disclose any help received and sources used.
- In an oral assessment, every student shall be able to present and answer questions about the entire assignment and solution.
Further information
Course room in Canvas
Offered by
Main field of study
Education cycle
Add-on studies
DD2431 Machine Learning
DD2434 Machine Learning, advanced course
DD2424 Deep learning in data science
DD2432 Artificial Neural Networks and Other Learning Systems
DD2423 Image Analysis and Computer Vision
DD2425 Robotics and Autonomous Systems
DD2429 Computational Photography
EL2320 Applied Estimation
Contact
Transitional regulations
The earlier examination LAB1 has been replaced by LAB2 and TEN2 has been replaced by TENQ.
Supplementary information
In this course, the EECS code of honor applies, see:
http://www.kth.se/en/eecs/utbildning/hederskodex