- The basics of the probabilistic method.
- Probabilistic modelling.
- Dimensionality reduction.
- Graphical models.
- Hidden Markov models.
- Expectation-Maximization.
- Variational Inference.
- Networks in variational inference.
DD2434 Machine Learning, Advanced Course 7.5 credits
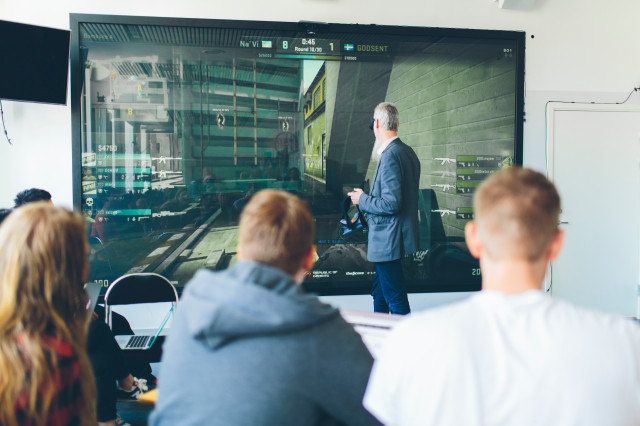
A second course in machine learning, giving a broadened and deepened introduction to the area.
About course offering
For course offering
Autumn 2024 mladv24 programme students
Target group
Open for all students from year 3 and for students admitted to a master's programme, as long as it can be included in your programme.
Part of programme
Master's Programme, Applied and Computational Mathematics, åk 1, Optional
Master's Programme, Applied and Computational Mathematics, åk 2, Optional
Master's Programme, Computer Science, åk 2, CSCS, Recommended
Master's Programme, Computer Science, åk 2, CSDA, Conditionally Elective
Master's Programme, Computer Simulations for Science and Engineering, åk 2, Conditionally Elective
Master's Programme, Cybersecurity, åk 1, Recommended
Master's Programme, Cybersecurity, åk 2, Recommended
Master's Programme, ICT Innovation, åk 1, DASC, Recommended
Master's Programme, ICT Innovation, åk 1, DASE, Recommended
Master's Programme, ICT Innovation, åk 2, DASC, Recommended
Master's Programme, ICT Innovation, åk 2, DASE, Recommended
Master's Programme, Industrial Engineering and Management, åk 1, MAIG, Conditionally Elective
Master's Programme, Information and Network Engineering, åk 2, Recommended
Master's Programme, Information and Network Engineering, åk 2, INF, Recommended
Master's Programme, Machine Learning, åk 1, Mandatory
Master's Programme, Mechatronics, åk 1, Conditionally Elective
Master's Programme, Mechatronics, åk 2, Conditionally Elective
Master's Programme, Systems, Control and Robotics, åk 1, LDCS, Conditionally Elective
Master's Programme, Systems, Control and Robotics, åk 1, RASM, Conditionally Elective
Master's Programme, Systems, Control and Robotics, åk 2, LDCS, Conditionally Elective
Master's Programme, Systems, Control and Robotics, åk 2, RASM, Conditionally Elective
Periods
P2 (7.5 hp)Duration
Pace of study
50%
Form of study
Normal Daytime
Language of instruction
English
Course location
KTH Campus
Number of places
Places are not limited
Planned modular schedule
Course memo
Course memo is not publishedSchedule
Link to scheduleApplication
For course offering
Autumn 2024 mladv24 programme students
Application code
52222
Contact
For course offering
Autumn 2024 mladv24 programme students
Contact
Jens Lagergren (jensl@kth.se)
Examiner
No information insertedCourse coordinator
No information insertedTeachers
No information insertedContent and learning outcomes
Course contents
Intended learning outcomes
After passing the course, the student should be able to
- explain and justify several important methods for machine learning
- give an account of several types of methods and algorithms that are used in the field of deterministic inference methods
- implement several types of methods and algorithms that are used in the field based on a high-level description
- extend and modify the methods that the course deals with
in order to be able to do a degree project in deterministic inference methods.
Literature and preparations
Specific prerequisites
- Knowledge and skills in Programming, 6 credits, corresponding to completed course DD1337/DD1310-DD1319/DD1321/DD1331/DD100N/ID1018.
- Knowledge in Linear Algebra, 7,5 credits, corresponding to completed course SF1624/SF1672/SF1684.
- Knowledge in Calculus in Several Variables, 7,5 credits, corresponding to completed course SF1626/SF1674.
- Knowledge in Probability Theory and Statistics, 6 credits, corresponding to completed course SF1910-SF1924/SF1935
and at least one of the following:
either
- Knowledge of basic Machine Learning, 7,5 credits, corresponding to completed course DD1420/DD2421/EL2810/EQ2341
or
- Knowledge of higher mathematics relevant to advanced Machine Learning, 15 credits, e.g. corresponding to completed courses SF2940 Probability Theory and SF2955 Computer Intensive Methods in Mathematical Statistics.
- Knowledge of basic computer science, 6 credits, corresponding to completed course DD1338/DD1320-DD1328/DD2325/ID1020/ID1021.
- Additional skills in independent software development, 12 credits, from completed courses in computer science, computer technology or numerical methods with laboratory elements that are not carried out in groups larger than two people. These courses are in addition to the above mentioned courses.
Active participation in a course offering where the final examination is not yet reported in Ladok is considered equivalent to completion of the course.
Registering for a course is counted as active participation.
The term 'final examination' encompasses both the regular examination and the first re-examination.
Recommended prerequisites
For KTH students, the recommended preparation is DD1420.
Also DD2421 and EL2810 are accepted as special eligibility requirements, but more time and effort may be required to complete the course.
Equipment
Literature
Examination and completion
If the course is discontinued, students may request to be examined during the following two academic years.
Grading scale
Examination
- HEM1 - Take-home exam, 3.5 credits, grading scale: A, B, C, D, E, FX, F
- PRO1 - Project assignment, 4.0 credits, grading scale: A, B, C, D, E, FX, F
Based on recommendation from KTH’s coordinator for disabilities, the examiner will decide how to adapt an examination for students with documented disability.
The examiner may apply another examination format when re-examining individual students.
Opportunity to complete the requirements via supplementary examination
Opportunity to raise an approved grade via renewed examination
Examiner
Ethical approach
- All members of a group are responsible for the group's work.
- In any assessment, every student shall honestly disclose any help received and sources used.
- In an oral assessment, every student shall be able to present and answer questions about the entire assignment and solution.
Further information
Course room in Canvas
Offered by
Main field of study
Education cycle
Add-on studies
Contact
Transitional regulations
TEN1 is replaced by HEM1 and LAB1 is replaced by PRO1.
Supplementary information
Grading criteria are made available when the course starts.
In this course, the EECS code of honor applies, see:
http://www.kth.se/en/eecs/utbildning/hederskodex