Innehåll:
• Introduction till Data Mining
• Frekventa Itemmängder
• Att hitta liknande enheter
• Klustring
• Recommender-system
• Mining Data Streams
• Dimensionalitetsreduktion
• Storskalig maskininlärning
• Översikt av aktuell forskning inom data mining och dess koppling till andra relevanta forskningsområden.
FID3016 Data mining 7,5 hp
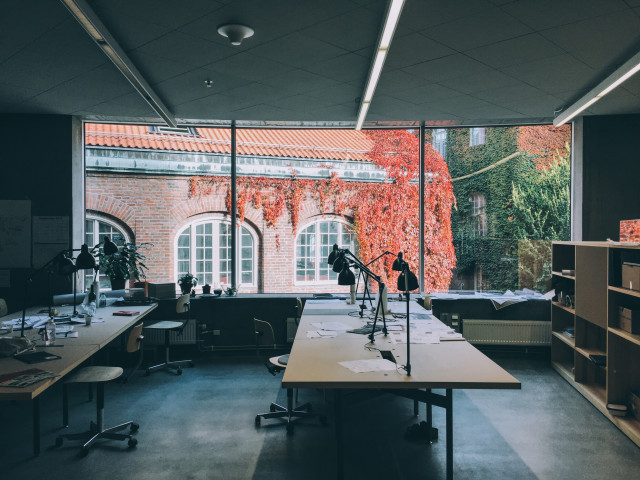
Information per kursomgång
Information för HT 2024 Start 2024-10-28 programstuderande
- Studielokalisering
KTH Kista
- Varaktighet
- 2024-10-28 - 2025-01-13
- Perioder
- P2 (7,5 hp)
- Studietakt
50%
- Anmälningskod
50918
- Undervisningsform
Normal Dagtid
- Undervisningsspråk
Engelska
- Kurs-PM
- Kurs-PM är inte publicerat
- Antal platser
Ingen platsbegränsning
- Målgrupp
- Ingen information tillagd
- Planerade schemamoduler
- [object Object]
- Schema
- Schema är inte publicerat
- Del av program
- Ingen information tillagd
Kontakt
Kursplan som PDF
Notera: all information från kursplanen visas i tillgängligt format på denna sida.
Kursplan FID3016 (VT 2019–)Innehåll och lärandemål
Kursinnehåll
Lärandemål
Kursen behandlar fundamenta inom data mining, data stream processing, och maskininlärningsalgoritmer för att analysera mycket stora datamängder. Vi använder plattformar för big data, såsom MapReduce, Spark och Apache Flink, för att implementera parallella algoritmer, och även beräkningssystem för data stream processing, såsom Storm och InfoSphere.
Efter denna kurs kommer studenterna att kunna utföra data mining på olika typer av data, t.ex. data av högre dimension, grafdata, och infinita/icke-avslutade data (data streams); liksom att programmera och bygga tillämpningar inom data-mining. De förväntas också att kunna lösa problem i praktiska tillämpningar, t.ex. recommender-system, associationsregler, länkanalys, och detektion av duplikat. Dessutom kommer de att behärska olika matematiska tekniker t.ex. linjär algebra, optimering, och dynamisk programmering. Dessutom ska studenten kunna beskriva och tillämpa aktuella forsknings trender i data mining (inklusive metoder, algoritmer, språkstöd och verktyg).
Kurslitteratur och förberedelser
Särskild behörighet
Rekommenderade förkunskaper:
Kännedom om begrepp och terminologi associerad med statistik, databassystem, och maskininlärning; en kurs om datastrukturer, algoritmer, och diskret matematik (som ID1020 Algoritmer och datastrukturer); en kurs i mjukvarusystem, software engineering, och programmeringsspråk; en kurs om att behandla, lagra and analysera massiva datamängder (som ID2221 Data-Intensive Computing).
Rekommenderade förkunskaper
Rekommenderade förkunskaper:
Kännedom om begrepp och terminologi associerad med statistik, databassystem, och maskininlärning; en kurs om datastrukturer, algoritmer, och diskret matematik (som ID1020 Algoritmer och datastrukturer); en kurs i mjukvarusystem, software engineering, och programmeringsspråk; en kurs om att behandla, lagra and analysera massiva datamängder (som ID2221 Data-Intensive Computing).
Utrustning
Kurslitteratur
Examination och slutförande
När kurs inte längre ges har student möjlighet att examineras under ytterligare två läsår.
Betygsskala
Examination
- EXA1 - Examination, 7,5 hp, betygsskala: P, F
Examinator beslutar, baserat på rekommendation från KTH:s handläggare av stöd till studenter med funktionsnedsättning, om eventuell anpassad examination för studenter med dokumenterad, varaktig funktionsnedsättning.
Examinator får medge annan examinationsform vid omexamination av enstaka studenter.
Övriga krav för slutbetyg
Godkänd skriftlig tentamina, godkända inlämningsuppgifter och godkänd tillämpning av nuvarande forskning (till exempel: användning i en forskningsartikel, forskningsrapport, eller forskningsprojekt).
Möjlighet till komplettering
Möjlighet till plussning
Examinator
Etiskt förhållningssätt
- Vid grupparbete har alla i gruppen ansvar för gruppens arbete.
- Vid examination ska varje student ärligt redovisa hjälp som erhållits och källor som använts.
- Vid muntlig examination ska varje student kunna redogöra för hela uppgiften och hela lösningen.