Viktiga ämnen i kursen inkluderar:
• Vad är maskininlärning?
• Beslutsfattande i en osäker värld
• Optimering
• Generalisering
• Probabilistiska metoder inom maskininlärning
• Informationsteori inom maskininlärning
• Geometri inom maskininlärning
• Kernel-metoder
• Neurala nätverk och djupinlärning
• Maskininlärning för datasyntes
• Interaktiv maskininlärning
• Ensemblemetoder
• Inlärningsteori
• Maskininlärning och omvärlden
DD1420 Maskininlärningens grunder 7,5 hp
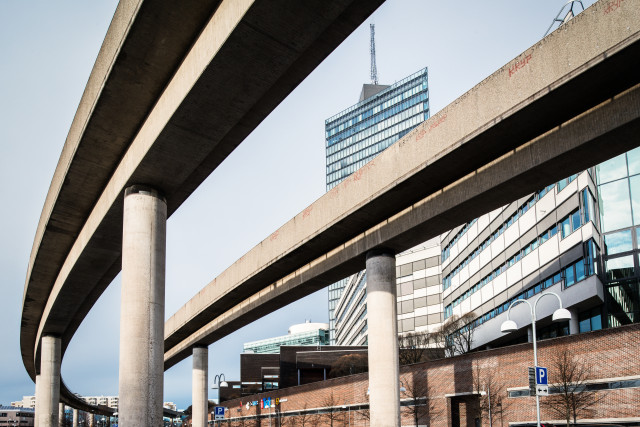
Denna kurs är tänkt som en första kurs i maskininlärning (ML) för studenter som tänker bli experter inom området. Kursen ger en bred överblick över teori och praktik i maskininläming från olika områden och perspektiv. Särskild vikt läggs på att lära ut kopplingar mellan fält och hur allting inom ML hänger samman, samt på "learning by doing". En väsentlig del av kursen involverar att lösa maskininlämingsproblem och jämföra lösningar, både på egen hand och tillsammans med andra blivande maskininlämingsexperter på KTH. Målet är att utrusta dig med en solid teoretisk och praktisk grund för att läsa avancerade maskininlämingskurser på KTH, samt att förbereda dig för en karriär inom maskininläming.
Om kursomgång
Gäller för kursomgång
HT 2024 GMLht23 programstuderande
Målgrupp
Sökbar för studenter i årskurs 3 och studenter på masterprogram, under förutsättning att kursen kan ingå i programmet.
Del av program
Masterprogram, cybersäkerhet, åk 1, Rekommenderad
Perioder
P1 (7,5 hp)Varaktighet
Studietakt
50%
Undervisningsform
Normal Dagtid
Undervisningsspråk
Engelska
Studielokalisering
KTH Campus
Antal platser
Ingen platsbegränsning
Planerade schemamoduler
Kurs-PM
Kurs-PM är inte publiceratSchema
Länk till SchemaKursval
Gäller för kursomgång
HT 2024 GMLht23 programstuderande
Anmälningskod
50244
Kontakt
Gäller för kursomgång
HT 2024 GMLht23 programstuderande
Examinator
Ingen information tillagdKursansvarig
Ingen information tillagdLärare
Ingen information tillagdInnehåll och lärandemål
Kursinnehåll
Lärandemål
Efter godkänd kurs ska studenten kunna:
- använda grundläggande begrepp, språk och notation som underbygger maskininlärning
- förklara de matematiska och statistiska mekanismerna inom vanliga maskininlärningsmetoder
- härleda och bevisa utvalda viktiga teoretiska resultat
- formulera och implementera lämpliga maskininlärningsmodeller för att lösa empiriska problem
- tolka modellens anpassning till data
- redogöra för styrkor och svagheter hos maskininlärningsmodeller/-tekniker och motivera gjorda val med utgångspunkt från datamaterialet
- identifiera relevant vetenskaplig litteratur, inklusive aktuella trender, och göra kritiska bedömningar av denna litteratur
- uppvisa kritiskt tänkande kring etiska och samhälleliga aspekter av maskininlärning och visa medvetenhet om aktuella framsteg inom dessa områden
i syfte att
- tydligt kunna definiera problem inom dataanalys
- formulera en ändamålsenlig lösning med maskininlärning och styrka denna lösning genom kritisk och kvantitativ utvärdering
- vara väl förberedd för att läsa avancerade kurser inom maskininlärning.
Kurslitteratur och förberedelser
Särskild behörighet
Slutförda kurser i samtliga av följande områden:
- Linjär algebra (SF1624, SF1672, SF1684 eller motsvarande)
- Flervariabelanalys (SF1626, SF1674 eller motsvarande)
- Sannolikhetslära och statistik (SF1912, SF1914-SF1924 eller motsvarande)
- Programmering (DD1310, DD1331, DD1337 eller motsvarande)
- Algoritmer och datastrukturer (DD1320, DD1321, DD1325, DD1327, DD1338, ID1020, ID1021 eller motsvarande)
Aktivt deltagande i kursomgång vars slutexamination ännu inte är Ladokrapporterad jämställs med slutförd kurs.
Den som är registrerad anses vara aktivt deltagande. Med slutexamination avses både ordinarie examination och det första omexaminationstillfället.
Rekommenderade förkunskaper
Utrustning
Kurslitteratur
Examination och slutförande
När kurs inte längre ges har student möjlighet att examineras under ytterligare två läsår.
Betygsskala
Examination
- INL1 - Inlämningsuppgift, 3,0 hp, betygsskala: A, B, C, D, E, FX, F
- PRO1 - Projektarbete, 1,5 hp, betygsskala: P, F
- TES1 - Quiz, 3,0 hp, betygsskala: A, B, C, D, E, FX, F
Examinator beslutar, baserat på rekommendation från KTH:s handläggare av stöd till studenter med funktionsnedsättning, om eventuell anpassad examination för studenter med dokumenterad, varaktig funktionsnedsättning.
Examinator får medge annan examinationsform vid omexamination av enstaka studenter.
Möjlighet till komplettering
Möjlighet till plussning
Examinator
Etiskt förhållningssätt
- Vid grupparbete har alla i gruppen ansvar för gruppens arbete.
- Vid examination ska varje student ärligt redovisa hjälp som erhållits och källor som använts.
- Vid muntlig examination ska varje student kunna redogöra för hela uppgiften och hela lösningen.
Ytterligare information
Kursrum i Canvas
Ges av
Huvudområde
Utbildningsnivå
Påbyggnad
Övrig information
Kurserna DD1420 och DD2421 överlappar innehållsmässigt.
Kurserna kan inte kombineras.