- Examples of applications of optimization and modelling training.
- Basic concepts and theory for optimization, in particular theory for convex problems.
- Linear algebra in Rn, in particular bases for the four fundamental subspaces corresponding to a given matrix, and LDLT-factorization of a symmetric positive semidefinite matrix.
- Linear optimization, including duality theory.
- Optimization of flows in networks.
- Quadratic optimization with linear equality constraints.
- Linear least squares problems, in particular minimum norm solutions.
- Unconstrained nonlinear optimization, in particular nonlinear least squares problems.
- Optimality conditions for constrained nonlinear optimization, in particular for convex problems.
- Lagrangian relaxation.
SF1811 Optimization 6.0 credits
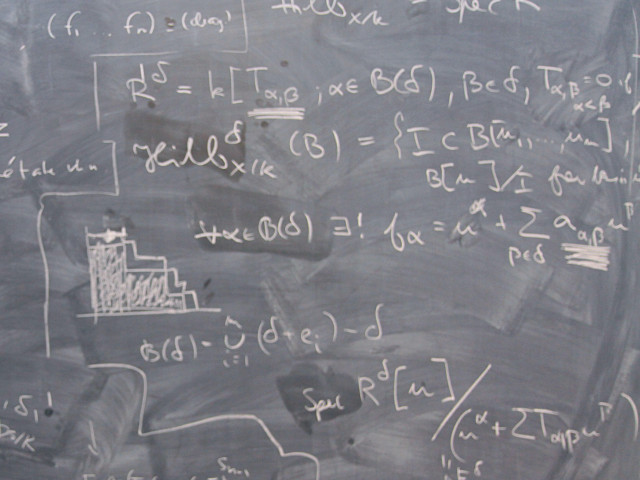
SF1811 is a basic course on optimization.
About course offering
For course offering
Autumn 2024 Start 28 Oct 2024 programme students
Target group
No information insertedPart of programme
Bachelor's Programme in Information and Communication Technology, åk 3, Recommended
Degree Programme in Energy and Environment, åk 3, HSS, Conditionally Elective
Degree Programme in Energy and Environment, åk 3, ITH, Mandatory
Degree Programme in Energy and Environment, åk 3, KEM, Conditionally Elective
Degree Programme in Energy and Environment, åk 3, MES, Conditionally Elective
Degree Programme in Energy and Environment, åk 3, MHI, Conditionally Elective
Degree Programme in Energy and Environment, åk 3, RENE, Conditionally Elective
Degree Programme in Energy and Environment, åk 3, SMCS, Conditionally Elective
Degree Programme in Energy and Environment, åk 3, SUE, Conditionally Elective
Degree Programme in Energy and Environment, åk 3, SUT, Conditionally Elective
Degree Programme in Engineering Mathematics, åk 3, Mandatory
Degree Programme in Engineering Physics, åk 3, Optional
Degree Programme in Industrial Technology and Sustainability, åk 3, Mandatory
Master's Programme, Applied and Computational Mathematics, åk 1, Optional
Master's Programme, Applied and Computational Mathematics, åk 2, Optional
Master's Programme, Computer Science, åk 2, CSDA, Recommended
Master's Programme, Machine Learning, åk 1, Conditionally Elective
Master's Programme, Machine Learning, åk 2, Conditionally Elective
Master's Programme, Systems, Control and Robotics, åk 1, Recommended
Master's Programme, Systems, Control and Robotics, åk 2, Recommended
Periods
P2 (6.0 hp)Duration
Pace of study
33%
Form of study
Normal Daytime
Language of instruction
English
Course location
KTH Campus
Number of places
Places are not limited
Planned modular schedule
Course memo
Course memo is not publishedSchedule
Link to scheduleApplication
For course offering
Autumn 2024 Start 28 Oct 2024 programme students
Application code
50177
Contact
For course offering
Autumn 2024 Start 28 Oct 2024 programme students
Contact
Anders Forsgren (andersf@kth.se)
Examiner
No information insertedCourse coordinator
No information insertedTeachers
No information insertedContent and learning outcomes
Course contents
Intended learning outcomes
After completing the course students should for a passing grade be able to
- Apply basic theory, concepts and methods, within the parts of optimization theory described by the course content, to solve problems
- Formulate simplified application problems as optimization problems and solve using software.
- Read and understand mathematical texts about for example, linear algebra, calculus and optimization and their applications, communicate mathematical reasoning and calculations in this area, orally and in writing in such a way that they are easy to follow.
For higher grades the student should also be able to
- Explain, combine and analyze basic theory, concepts and methods within the parts of optimization theory described by the course content.
Literature and preparations
Specific prerequisites
Completed course in SF1624 Linear algebra and geometry or SF1672 Linear Algebra.
Completed course in SF1626 Calculus in several variables or SF1674 Multivariable Calculus.
Completed course in Numerical analysis, SF1511, SF1519, SF1545 or SF1546.
Recommended prerequisites
Equipment
Literature
The literature is published on the course webpage no later than four weeks before the course starts.
Examination and completion
If the course is discontinued, students may request to be examined during the following two academic years.
Grading scale
Examination
- INL1 - Home assignment, 2.0 credits, grading scale: P, F
- TEN2 - Exam, 4.0 credits, grading scale: A, B, C, D, E, FX, F
Based on recommendation from KTH’s coordinator for disabilities, the examiner will decide how to adapt an examination for students with documented disability.
The examiner may apply another examination format when re-examining individual students.
The examiner decides, in consultation with KTHs Coordinator of students with disabilities (Funka), about any customized examination for students with documented,lastingdisability. The examiner may allow another form of examination for reexamination of individual students.
Opportunity to complete the requirements via supplementary examination
Opportunity to raise an approved grade via renewed examination
Examiner
Ethical approach
- All members of a group are responsible for the group's work.
- In any assessment, every student shall honestly disclose any help received and sources used.
- In an oral assessment, every student shall be able to present and answer questions about the entire assignment and solution.
Further information
Course room in Canvas
Offered by
Main field of study
Education cycle
Add-on studies
SF2812 Applied Linear Optimization, SF2822 Applied Nonlinear Optimization