- Ickelinjär optimering utan bivillkor: optimalitetsvillkor, Newtonmetoder, kvasi-Newtonmetoder, konjugerade gradientmetoder.
- Ickelinjär optimering med bivillkor: optimalitetsvillkor, kvadratisk programmering, sekvensiell kvadratisk programmering, barriärmetoder, primal-duala inrepunktsmetoder.
- Semidefinit programmering med inrepunktsmetoder.
- Konvexitet och konvexa relaxeringar.
SF2822 Tillämpad ickelinjär optimering 7,5 hp
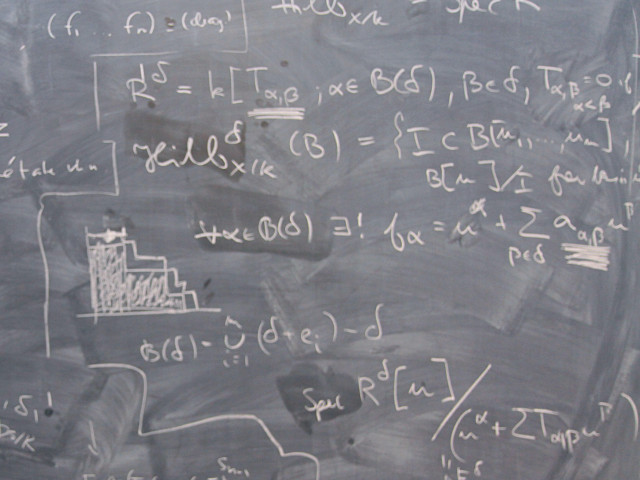
Om kursomgång
Gäller för kursomgång
VT 2025 Start 2025-03-17 programstuderande
Målgrupp
Sökbar för alla program under förutsättning att kursen kan ingå i programmet.
Del av program
Masterprogram, datorsimuleringar inom teknik och naturvetenskap, åk 1, Villkorligt valfri
Masterprogram, elkraftteknik, åk 1, Rekommenderad
Masterprogram, flyg- och rymdteknik, åk 1, Valfri
Masterprogram, flyg- och rymdteknik, åk 1, SYS, Obligatorisk
Masterprogram, industriell ekonomi, åk 1, OSYT, Villkorligt valfri
Masterprogram, information och nätverksteknologi, åk 1, Rekommenderad
Masterprogram, matematik, åk 1, Valfri
Masterprogram, systemteknik och robotik, åk 1, LDCS, Villkorligt valfri
Masterprogram, systemteknik och robotik, åk 2, LDCS, Villkorligt valfri
Masterprogram, tillämpad matematik och beräkningsmatematik, åk 1, Valfri
Masterprogram, tillämpad matematik och beräkningsmatematik, åk 1, OPST, Villkorligt valfri
Masterprogram, tillämpad matematik och beräkningsmatematik, åk 2, OPST, Villkorligt valfri
Perioder
P4 (7,5 hp)Varaktighet
Studietakt
50%
Undervisningsform
Normal Dagtid
Undervisningsspråk
Engelska
Studielokalisering
KTH Campus
Antal platser
Ingen platsbegränsning
Planerade schemamoduler
Kurs-PM
Kurs-PM är inte publiceratSchema
Schema är inte publiceratKursval
Gäller för kursomgång
VT 2025 Start 2025-03-17 programstuderande
Anmälningskod
60422
Kontakt
Gäller för kursomgång
VT 2025 Start 2025-03-17 programstuderande
Kontaktperson
Anders Forsgren (andersf@kth.se)
Examinator
Ingen information tillagdKursansvarig
Ingen information tillagdLärare
Ingen information tillagdInnehåll och lärandemål
Kursinnehåll
Lärandemål
Efter godkänd kurs ska studenten kunna:
- Tillämpa teori, begrepp och metoder inom de delar av optimeringslära som beskrivs av kursinnehållet för att lösa problem.
- Modellera, formulera och analysera förenklade tillämpningsproblem som optimeringsproblem och lösa med tillhandahållen programvara.
- Samarbeta med andra studenter och visa förmåga att presentera muntligt och skriftligt
För att uppnå högsta betyg ska studenten dessutom kunna följande:
- Kombinera och förklara kursens metoder samt
- Tillämpa och förklara kursens teori och begrepp på de tillämpningsproblem som ingår.
Kurslitteratur och förberedelser
Särskild behörighet
- Engelska B / Engelska 6
- Slutförd grundkurs i Optimeringslära (SF1811, SF1861 eller motsvarande)
- Slutförd grundkurs i Matematisk statistik (SF1914, SF1918, SF1922 eller motsvarande)
- Slutförd grundkurs i Numerisk analys (SF1544, SF1545 eller motsvarande)
- Slutförd grundkurs i Differentialekvationer (SF1633, SF1683 eller motsvarande)
Rekommenderade förkunskaper
En slutförd fortsättningskurs i numerisk analys.
Utrustning
Kurslitteratur
Anges vid kursstart. Preliminär litteratur:
Linear and Nonlinear Programming av S.G.Nash och A.Sofer, McGraw-Hill, samt kompletterande material från institutionen.
Examination och slutförande
När kurs inte längre ges har student möjlighet att examineras under ytterligare två läsår.
Betygsskala
Examination
- PRO1 - Projektuppgift 1, 1,5 hp, betygsskala: A, B, C, D, E, FX, F
- PRO2 - Projektuppgift 2, 1,5 hp, betygsskala: A, B, C, D, E, FX, F
- TEN1 - Tentamen, 4,5 hp, betygsskala: A, B, C, D, E, FX, F
Examinator beslutar, baserat på rekommendation från KTH:s handläggare av stöd till studenter med funktionsnedsättning, om eventuell anpassad examination för studenter med dokumenterad, varaktig funktionsnedsättning.
Examinator får medge annan examinationsform vid omexamination av enstaka studenter.
Möjlighet till komplettering
Möjlighet till plussning
Examinator
Etiskt förhållningssätt
- Vid grupparbete har alla i gruppen ansvar för gruppens arbete.
- Vid examination ska varje student ärligt redovisa hjälp som erhållits och källor som använts.
- Vid muntlig examination ska varje student kunna redogöra för hela uppgiften och hela lösningen.