Course memo Autumn 2023
Course presentation
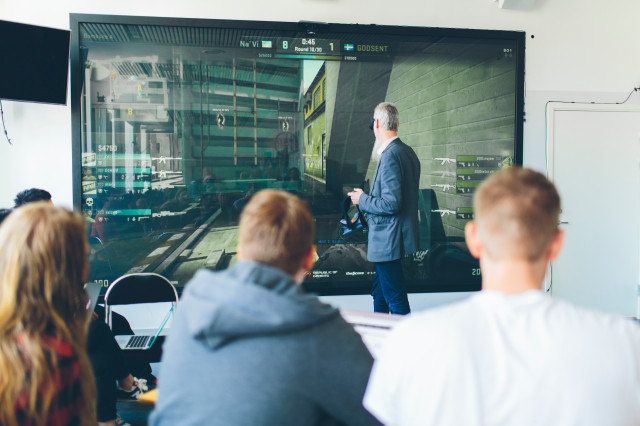
Headings denoted with an asterisk ( * ) is retrieved from the course syllabus version Spring 2021
Content and learning outcomes
Course contents
The course is intended for both undergraduate and graduate students in computer science and related fields such as engineering and statistics The course addresses the question how to enable computers to learn from past experiences It introduces the field of machine learning describing a variety of learning paradigms, algorithms, theoretical results and applications. It introduces basic concepts from statistics, artificial intelligence, information theory and probability theory in so far they are relevant to machine learning
The following topics in machine learning and computational intelligence are covered in detail
- nearest neighbour classifier
- decision trees
- bias and the trade-off of variance
- regression
- probabilistic methods
- Bayesian learning
- support vector machines
- artificial neural networks
- ensemble methods
- dimensionality reduction
- subspace methods.
Intended learning outcomes
After passing the course, the student should be able to
- describe the most important algorithms and the theory that constitutes the basis for machine learning and artificial intelligence
- explain the principle for machine learning and how the algorithms and the methods can be used
- discuss advantages with and limitations of machine learning for different applications
in order to be able to identify and apply appropriate machine learning technique for classification, pattern recognition, regression and decision problems.
Preparations before course start
Literature
No information insertedSupport for students with disabilities
Students at KTH with a permanent disability can get support during studies from Funka:
Examination and completion
Grading scale
A, B, C, D, E, FX, F
Examination
- LAB1 - Laboratory Work, 3.5 credits, Grading scale: P, F
- TEN1 - Examination, 4.0 credits, Grading scale: A, B, C, D, E, FX, F
Based on recommendation from KTH’s coordinator for disabilities, the examiner will decide how to adapt an examination for students with documented disability.
The examiner may apply another examination format when re-examining individual students.
The exam is written.
Opportunity to raise an approved grade via renewed examination
Grade raising is allowed.
Ethical approach
- All members of a group are responsible for the group's work.
- In any assessment, every student shall honestly disclose any help received and sources used.
- In an oral assessment, every student shall be able to present and answer questions about the entire assignment and solution.
Further information
No information inserted
Contacts
Course Coordinator
Teachers
Teacher Assistants
Examiner
Round Facts
Start date
28 Aug 2023
Course offering
- MLHT23 Autumn 2023-50342
Language Of Instruction
English