Mats Persson new assistant professor in photon-counting spectral x-ray computed tomography
NEW DIGITALISATION RESEARCHERS
The new KTH faculty member Mats Persson was appointed assistant professor at the Department of Physics, in the Physics of Medical Imaging Division, in 2020. After receiving his PhD from KTH in 2016 he has been a postdoc at Stanford University and at the GE Research Center in Niskayuna, New York. His research is focused on developing data-processing and reconstruction methods for photon-counting spectral x-ray computed tomography.
What is your research area?
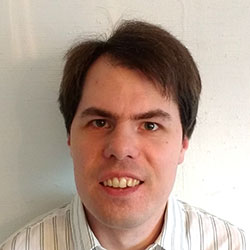
“My research is centered on medical x-ray imaging, in particular photon-counting x-ray computed tomography. After previously working with development of new x-ray detector hardware, I have recently moved into the field of image reconstruction where the emerging deep-learning-based image reconstruction methods are right now bringing about a revolution in how we generate images from measured data. I am studying how deep neural networks trained on large sets of x-ray images can be combined with an understanding of the physics of x-ray imaging in order to generate computed tomography images with much improved accuracy and diagnostic quality,” Mats Persson says.
If you are looking for research collaborators, what competence is important?
“Medical imaging is a truly multidisciplinary field, and we therefore need to collaborate with a diverse set of experts in academia, industry and healthcare. In particular, we are always on the lookout for both medical specialists who can help us understand the clinical applications of this technology and experts in mathematics and AI who can help us solve the many challenges related to developing deep-learning-based image reconstruction algorithms.”
What are the large research challenges in your research area and why?
“One of the largest research challenges in the field of medical imaging right now is to understand how the new AI-based image reconstruction methods actually work. Instead of viewing the deep neural network as a ‘black box’, we would like to understand how the properties of the output image is related to the choice of neural network model, the input data and the image dataset used for training. This can facilitate the adoption of the new technology in the clinic. For example, providing the user not only with an image of the interior of the body but also an estimate of how certain the measurements are will help building trust in the new technology and allow healthcare practitioners to make more informed decisions.”
“Another research challenge is to collect and distribute the large sets of image data that is needed to train neural-network-based reconstruction methods, given that a single x-ray examination can be several gigabytes large and that concerns related to patient privacy must be addressed appropriately.”
Tell us more about one of your research results and why you picked it?
“In a collaboration between our research group and the Department of Mathematics at KTH, we have demonstrated that photon-counting computed tomography with a deep-learning based image reconstruction algorithm can be used to separate three different materials in photon-counting x-ray computed tomography images: soft tissue, bone and iodinated contrast agent. This is hard to perform without having both an energy-resolving photon-counting computed tomography scanner and a deep-learning based image reconstruction algorithm, demonstrating the interplay of advances in both hardware and software. This finding can have important implications for example for diagnosing cardiovascular disease, where it is important to visualize both the blood with contrast agent and calcified plaques at the same time.”
Lastly, what do you like with Sweden, Stockholm and KTH?
“A good thing with doing applied research in Sweden is the favorable climate for entrepreneurship, which has allowed us to commercialize our research through a startup company. It is also good that KTH supports close collaboration between academia and industry, which makes it possible to bring new technology to the benefit of the general public in a way that may be hard in other places. We also have a very strong ecosystem for biomedical engineering here in Stockholm, for example within MedTechLabs, our center that brings together researchers from KTH, Karolinska Institutet and Karolinska University Hospital to solve real-world problems in health care.”