GeneDisco Challenge
for machine learning-enabled Drug discovery

We talked to Stefan Bauer who is organising a Machine Learning for Drug Discovery Workshop and GeneDisco Challenge, Friday 29 April.
What excites you about these challenges?
"We are at a pivotal moment in healthcare: unprecedented scientific and technological progress in biology over the past two decades bears the promise of radically transforming how we develop treatments and provide care to patients. Yet, drug discovery has become an increasingly challenging endeavour: the success rate of developing new therapeutics has been historically low, but this rate has been steadily declining."
"The average cost of bringing a new drug to market is twice as high as just a decade earlier. Machine learning-based approaches present a unique opportunity to address this challenge. Yet, applying machine learning techniques to the problem of early-stage drug discovery benchmarks to make systematic progress has been missing. Only very privileged labs in industry and academia had the possibility for ML-driven drug discovery while the challenge opens up the field and lets everyone participate."
What do you look most forward to around the events?
"Joint with the GeneDisco Challenge for machine learning-enabled Drug discovery, we organise a workshop on the same topic. The workshop will feature talks from leading researchers and pioneers from academia and industry, fireside chats, and expert panel discussions. Hopefully, this will result in actionable and transnational insights and open up the field to many participants."
What do you think the participant will learn in the Machine Learning for Drug Discovery Workshop discussions?
"Machine learning can enable and help at various stages of the drug discovery process. During the discussions at the workshop, the participants should see in which areas, e.g. from molecule optimisation to experiment design and see in poster sessions and talks what other members both from industry and academia are working on."
What would the ultimate or dream result be from the Machine Learning for Drug Discovery Workshop?
"We aim to bring together the community to discuss cutting-edge research in machine learning-enabled drug discovery for the workshop."
"While there has been growing interest and pioneering work in the machine learning (ML) community over the past decade, the broader community's specific challenges posed by drug discovery are mainly unknown. The ambition of the workshop is to federate the community interested in this application domain where
i) ML can have a significant positive impact for the benefit of all and
ii) the application domain can drive ML method development through novel problem settings, benchmarks and testing grounds at the intersection of many subfields ranging from representation, active and reinforcement learning to causality and treatment effects."
What is the GeneDisco Challenge?
"With billions of potential hypotheses to test, the experimental design space for in vitro genetic experiments is exceptionally vast. The available practical capacity - even at the most prominent research institutions in the world - pales concerning the size of this biological hypothesis space."
"Machine learning methods, such as active and reinforcement learning, could aid in optimally exploring the vast biological area by integrating prior knowledge from various information sources. However, there exist no standardised benchmarks and data sets for this challenging task. To solve this problem, we created GeneDisco, a benchmark suite for evaluating active learning algorithms for experimental design in drug discovery."
"GeneDisco contains a curated set of multiple publicly available experimental data sets and open-source implementations of state-of-the-art active learning policies for experimental design and exploration. Participants of the GeneDisco challenge can use the benchmark to contribute to systematic progress in using machine learning for early-stage drug discovery and target validation."
Why is it important to participate in GeneDisco Challenge?
"Machine learning methods, such as active and reinforcement learning, could potentially aid in optimally exploring the space of genetic interventions by prioritising experiments that are more likely to yield mechanistic insights of therapeutic relevance."
"Given the lack of openly accessible curated experimental benchmarks, there does not yet exist to date a concerted effort to leverage the machine learning community for advancing research in this crucial domain."
"Each participant has the chance to contribute to benchmarking and the new proposal of algorithms to extend machine learning-enabled drug discovery. More participants in the GeneDisco challenge will hopefully lead to a diverse set of proposed approaches, one of which might outperform the other methods consistently. If implemented at one of the big pharmaceutical companies, an increased success rate of 1 per cent can lead to tens or hundreds of new drugs every year."
In a couple of years, if you look back on this occasion and say, "It was on the GeneDisco Challenge 2022 that we experienced this fantastic outcome of worldwide importance."
What would that be?
"With the GeneDisco challenge, we hopefully provide the needed benchmark to test and advance machine learning-based approaches for early-stage drug discovery. In the best case, we find techniques that work reliably across all benchmark settings, which could lead to data-driven strategies that introduce additional hundreds of new and potentially life-changing therapeutic options for patients every year in the future."
"By establishing these benchmarks and organising community challenges, we open up the field of machine learning-enabled drug discovery and allow many more students, especially from less highly ranked universities, to participate in state-of-the-art and collaborative research."
More information:
Related news
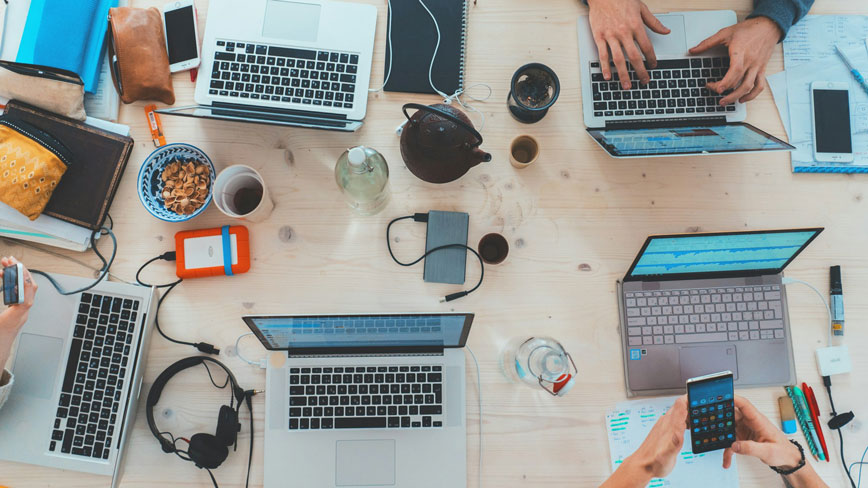
New algorithm makes machine learning faster and more accurate
The algorithm can improve the way smart devices in homes and workplaces work together. By reducing the frequency with which devices need to talk to a server while seamlessly handling different data be...
Read the articleFour scientists on the future of AI
Research in Artificial Intelligence was in focus when researchers and partners from Saab and Ericsson gathered for two days at CASTOR Software Days to discuss the research in the field.
Read the articleFrustration over parking fines led to action
A frustration with parking fines led to the development of a parking app that can read signs and help avoid fines. Now KTH students Maximillian Claesson, Industrial Engineering and Management, and Zak...
Read the article