Improved treatment of tumors with novel image tools
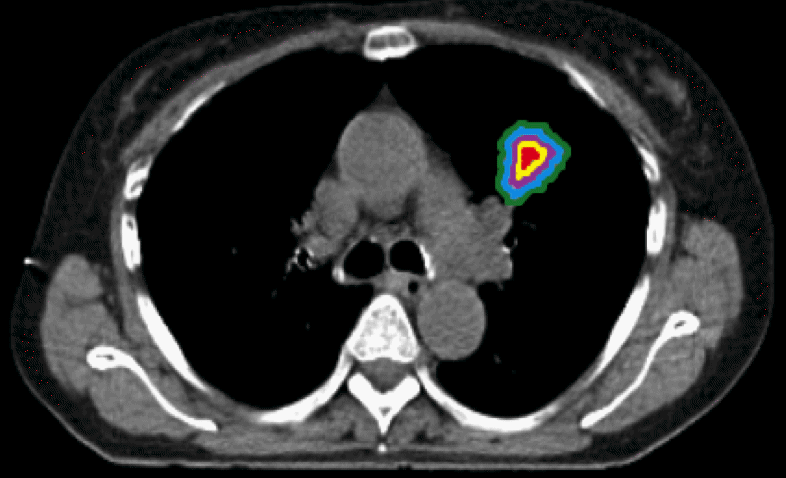
Description (background and methods)
For both surgery and radiotherapy, clinicians need exact information about the extent of the tumor and of structures that must not be damaged. With a modern CT or MRI examination consisting of hundreds of images (obtained with different modalities or sequences), it is not realistic in clinical routine to do this manually. Thus, new automated methods for segmentation (delineation by identifying the boundary) are needed. In particular, when evaluating whether a tumor grows or shrinks during treatment, reliable segmentation methods are crucial. In this project, new segmentation techniques based on machine learning techniques, such as support vector machines, decision forests and deep learning (U-Net), are being developed and tested in available datasets of brain tumors, lung cancer and other types of tumors. The segmentation methods are compared to conventional manual segmentation, and agreement between automated and manual segmentation will be related to the variation between human observers. For future clinical applications, we correlate the findings with long-term clinical outcome.
(Preliminary) results and publications
Buizza G, Toma-Dasu I, Lazzeroni M, Paganelli C, Riboldi M, Chang Y, Smedby Ö, Wang C. Early Tumor Response Prediction for Lung Cancer Patients Using Novel Longitudinal Pattern Features from Sequential PET/CT Image Scans. Physica Medica: European Journal of Medical Physics 2018;54:21–29 dx.doi.org/10.1016/j.ejmp.2018.09.003
Astaraki M, Wang CL, Buizza G, Toma-Dasu I, Lazzeroni M, Smedby Ö. Early Survival Prediction in Non-Small Cell Lung Cancer from PET/CT Images Using an Intra-Tumor Partitioning Method. Physica Medica: European Journal of Medical Physics 2019;6058–65 dx.doi.org/10.1016/j.ejmp.2019.03.024
Astaraki M, Wang C, Buizza G, Toma-Dasu I, Lazzeroni M, Smedby Ö. Early survival prediction in non-small cell lung cancer with PET/CT size aware longitudinal pattern. Radiotherapy and Oncology. 2019;133:S208-S209. dx.doi.org/10.1016/S0167-8140(19)30826-6
Astaraki M, Toma-Dasu I, Smedby Ö, Wang C. Normal Appearance Autoencoder for Lung Cancer Detection and Segmentation. In: Shen D. et al. (eds) Medical Image Computing and Computer Assisted Intervention – MICCAI 2019. MICCAI 2019. Lecture Notes in Computer Science, vol 11769. Springer, Cham doi.org/10.1007/978-3-030-32226-7_28
Wang C, Smedby Ö. Automatic Brain Tumor segmentation Using 2.5D U-nets. MICCAI workshop on multimodal brain tumor segmentation (BRATS) challenge, Québec, 2017. p. 292-96.
Collaborations
- Medical radiation science, KI
- Oncology, KI
- Center for Medical Image Science and Visualization (CMIV), Linköping University
- Neurosurgical dept., Linköping University Hospital
- Elekta Instrument AB
- ContextVision AB
Thesis projects
Mehdi Astaraki, doctoral dissertation project.